Food, Global Health Reading time 3 min
Major breakthrough in biology: a hybrid generative AI designs new molecules
PRESS RELEASE - Predicting the structure of proteins, creating new ones. Protein design is a rapidly evolving field of research owing to the possibilities offered by AI, with the possibility of designing new proteins and enzymes with direct applications in health and the environment. Researchers in mathematics at INRAE have developed an AI combining learning and reasoning. This hybrid AI is thus capable of designing proteins according to rules learned through deep learning, but also derived from physics or made explicit by designers. Results that contribute to the range of protein design methods, presented in Nature Reviews Methods Primers.
Published on 03 March 2025
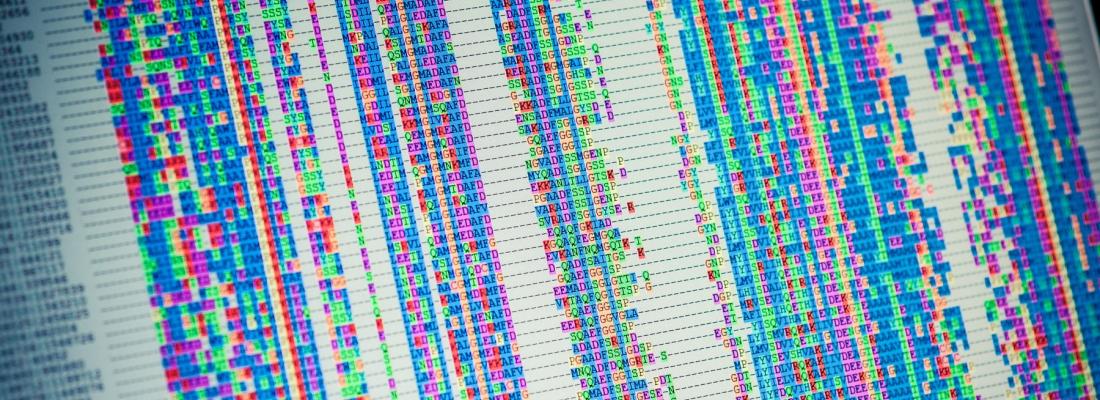
Proteins play a central role in the development and functioning of living organisms. From a chemical point of view, an unfolded protein resembles a string of pearls, each pearl being an amino acid (there are 20 different amino acids in living organisms). Depending on the type and number of amino acids, the protein acquires a specific shape in space. This “folding” phenomenon determines the function of the protein, such as the transport of molecules in the blood, enzymatic digestion or the reception and transmission of signals. In all, our bodies contain around 20,000 different types of proteins. The transition from an amino acid sequence to a 3D form is a highly complex phenomenon, and understanding it is a major research challenge, particularly for studying certain diseases caused by misfolded proteins, including neurodegenerative diseases such as Alzheimer's and Parkinson's.
One area of research is currently advancing at a phenomenal pace: computational protein design, as recently highlighted by the 2024 Nobel Prize in Chemistry. The ability to design completely new proteins for a specific purpose truly opens up revolutionary possibilities in the fields of health and the environment.
As a result of a long-standing collaboration between artificial intelligence researchers and molecular modellers[1], INRAE has contributed to these advances through design methods based on hybrid artificial intelligence, combining learning and reasoning, already used to design various new, functional proteins that have been experimentally characterised.
Historically, physics had allowed for a partially satisfactory reduction of the protein design problem to a difficult mathematical optimisation one. But AI has disrupted this approach. The chemical composition of a protein is defined by a simple text (its sequence) which also defines its final 3D shape. Natural language generative AIs (such as ChatGPT, Gemini or Llama) were therefore immediately adapted to the language of natural protein sequences while those targeting the generation of 2D images (like DALL-E, Midjourney or Flux) were similarly extended to generate new protein structures. The main challenge is guiding these AIs to create the protein with the desired capabilities.
A hybrid AI capable of learning to play logic games such as Sudoku simply by observing solved grids
The methods developed by INRAE researchers combine 2 families of AI tools: deep learning and automated reasoning.
Deep learning is used to extract the rules that govern protein design, by exploiting the sequences and structures of natural proteins that have been accumulated over decades by biophysicists in the Protein Data Bank. Automated reasoning is used for its ability to combine these learned rules with fundamental laws of physics and the designer's instructions, in order to very rapidly identify different proteins that meet these requirements among the exponential universe of possible proteins.
Together they form a so-called neuro-symbolic generative AI, capable of designing proteins that precisely comply with the designers' instructions. The researchers have shown that this architecture is also capable of learning to play logic games such as Sudoku perfectly, without explicitly being taught the rules, by simply observing solved grids.
These methods considerably democratise the ability to design new proteins, even if mastering the design process itself still requires a great deal of expertise.
[1] In particular through the involvement in projects run by ANITI (Artificial and Natural Intelligence Toulouse Institute).
Reference
Albanese K.I., Barbe S., Tagami S., Woolfson D., Schiex, T. (2025). Computational Protein Design. Nature Reviews Methods Primers, DOI: https://doi.org/10.1038/s43586-025-00383-1