Agroecology Reading time 3 min
Discriminating pathological, reproductive or stress conditions in cows using machine learning on sensor-based activity data
Machine learning on time series can detect and discriminate several conditions of cow impaired welfare and could thus be implemented in Precision livestock farming (PLF) systems to facilitate herd management.
Published on 31 March 2023
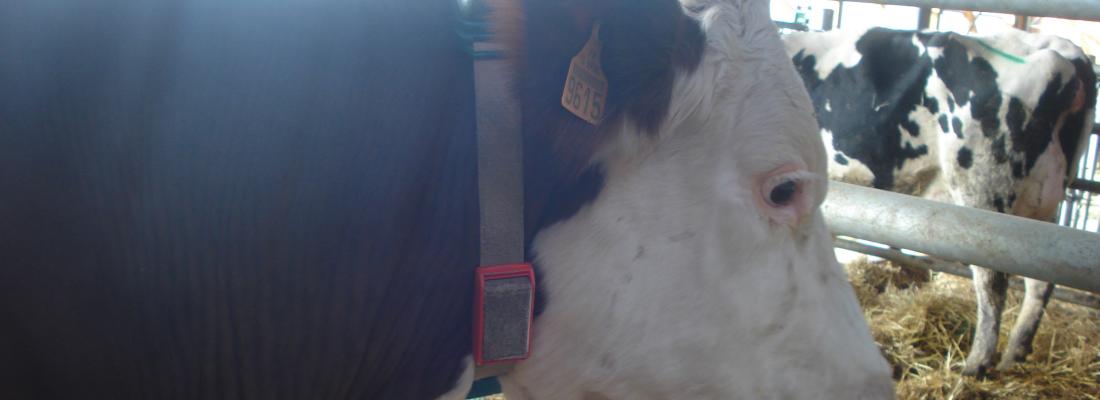
Precision livestock farming (PLF) technologies help to detect animals experiencing specific conditions such as illness, stress, or oestrus. PLF systems either detect one or more conditions at a time, or several conditions together but without distinguishing them.
The aim of this work was to detect and classify a wide range of conditions of health, stress and reproduction based on cow daily activity using machine learning on time series data derived from sensors. We used five datasets (120,000 cow*days) from experimental or commercial farms, with 28 to 300 Holstein cows per dataset and observations lasting from 1 to 12 months. Caretakers checked the animals every day and recorded any specific cow condition related to disease (lameness, mastitis, acidosis, accident, and so on), oestrus, calving, as well as potential stress due to mixing or handling. We labelled a number of days before and after the specific condition during which the behaviour might be altered. Sensors (location systems or accelerometers) captured the per-hour duration of key cow activities - eating, resting, in alleys – that served to compute the activity level. The distribution of the activity level in each 24 h time series was described by 21 time-domain or frequency-domain features. We then ran the random forest algorithm to relate the features of the time series to animal condition (1000 trees; 10 random splits of 70% train / 30% test subsets; oversampling applied on train subsets to reduce the imbalance between the occurrences of cow conditions). The most important features in the random forest algorithm were, in descending order: maximum, 90% quantile, Fourier harmonics 2 and 1, 50% and 75% quantiles, Fourier harmonics 3 and 4.
This approach correctly classified over 90% of the control series (i.e. with no specific condition) and classified 24% of the specific condition-labelled series as non-control series. The condition-labelled series can be confused with control series and to a lesser extent with series labelled disturbances or oestrus. There was 56%–86% probability of successfully detecting at least one 24 h series around a disease, oestrus, or calving. The detection can occur 1–2 days before the day caretakers noticed the event.
This study shows that machine learning on time series can detect and discriminate several conditions of cow impaired welfare and could thus be implemented in PLF systems to facilitate herd management. The sensitivity of the method must be improved before it can be spread out on farms.
Lardy, R.; Ruin, Q.; Veissier, I., 2023. Discriminating pathological, reproductive or stress conditions in cows using machine learning on sensor-based activity data. Computers and Electronics in Agriculture, 204: 10 http://dx.doi.org/10.1016/j.compag.2022.107556
Wagner N., Mialon MM, Sloth KH, Lardy R., Ledoux D., Silberberg M., De Boyer Des Roches A., Veissier I., 2021. Detection of changes in the circadian rhythm of cattle in relation to disease, stress, and reproductive events. Methods, 186 :14-21. http://doi.org/10.1016/j.ymeth.2020.09.003